Hungarian AI Researchers Abroad Gather in Hungary for MILAB's 2024 Machine Learning Days
The Hungarian Machine Learning Days, organised by the Artificial Intelligence National Laboratory (MILAB), attracted a remarkable turnout of world-renowned researchers of Hungarian origin working abroad, along with many PhD students. The summer event provided an excellent opportunity for researchers from abroad and in Hungary to collaborate on addressing current issues in the field.
For the second time, MILAB, coordinated by HUN-REN SZTAKI, organised the conference to provide machine learning researchers spending their summer break in Hungary with the opportunity to interact with each other, the domestic community, and students, and to present their findings. As András Benczúr, head of the SZTAKI Artificial Intelligence Laboratory and organiser of the event, told the HUN-REN portal, the discussions centred on understanding why machine learning researchers often achieve greater success abroad and how their experiences could be utilised in Hungary. The aim was also to explore ways to support the Hungarian community through both informal and formal programmes, including exchange and scholarship opportunities.
This year's event featured several PhD students of Hungarian origin from abroad, alongside researchers from the University of Warwick. In addition, researchers from institutions such as Google, DeepMind, Cambridge and Stanford Universities, ETH Zürich, and AIT Austria also gave presentations during the three-day conference.
With an increasing number of non-Hungarian-speaking students and researchers working in Hungary, the official language of the conference was English, while informal discussions were primarily conducted in Hungarian.
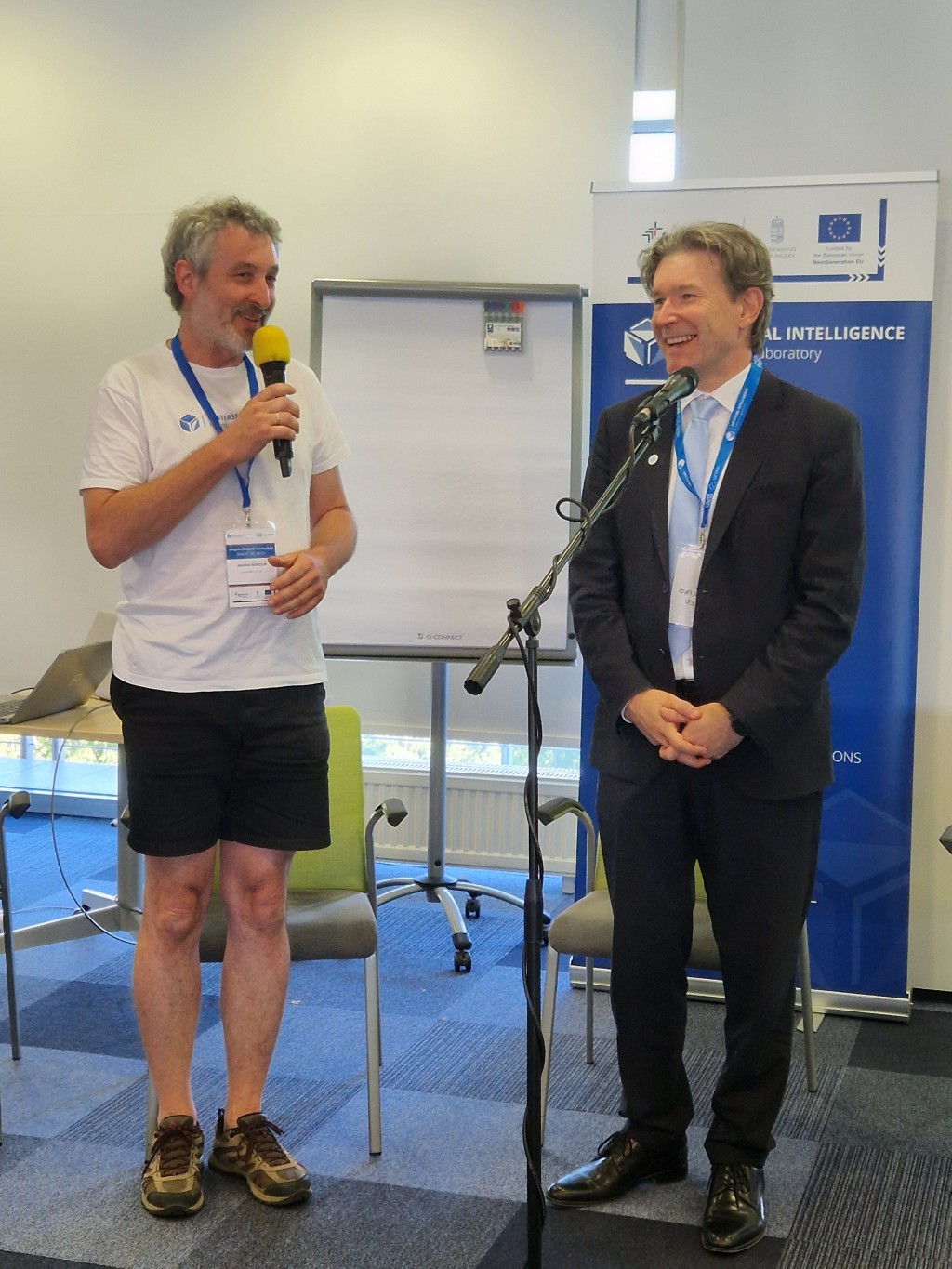
In his welcome speech, Roland Jakab, CEO of HUN-REN, discussed the AI Ambassador Programme, an initiative by the HUN-REN Headquarters aimed at integrating AI tools into all fields of science. Dedicated AI Ambassadors at research sites provide ongoing support to researchers in leveraging the potential of AI. Launched in 2024, the initiative offers researchers workshops, courses, hackathons, and training in the theory and practice of machine learning algorithms. It also provides professional assistance in selecting the right models, developing research plans, and advancing projects through the approval and implementation phases. The CEO also introduced the recently launched, state-funded Research Grant Hungary programme, which invites leading international researchers, including Hungarian scientists working abroad, to carry out their projects in Hungary.
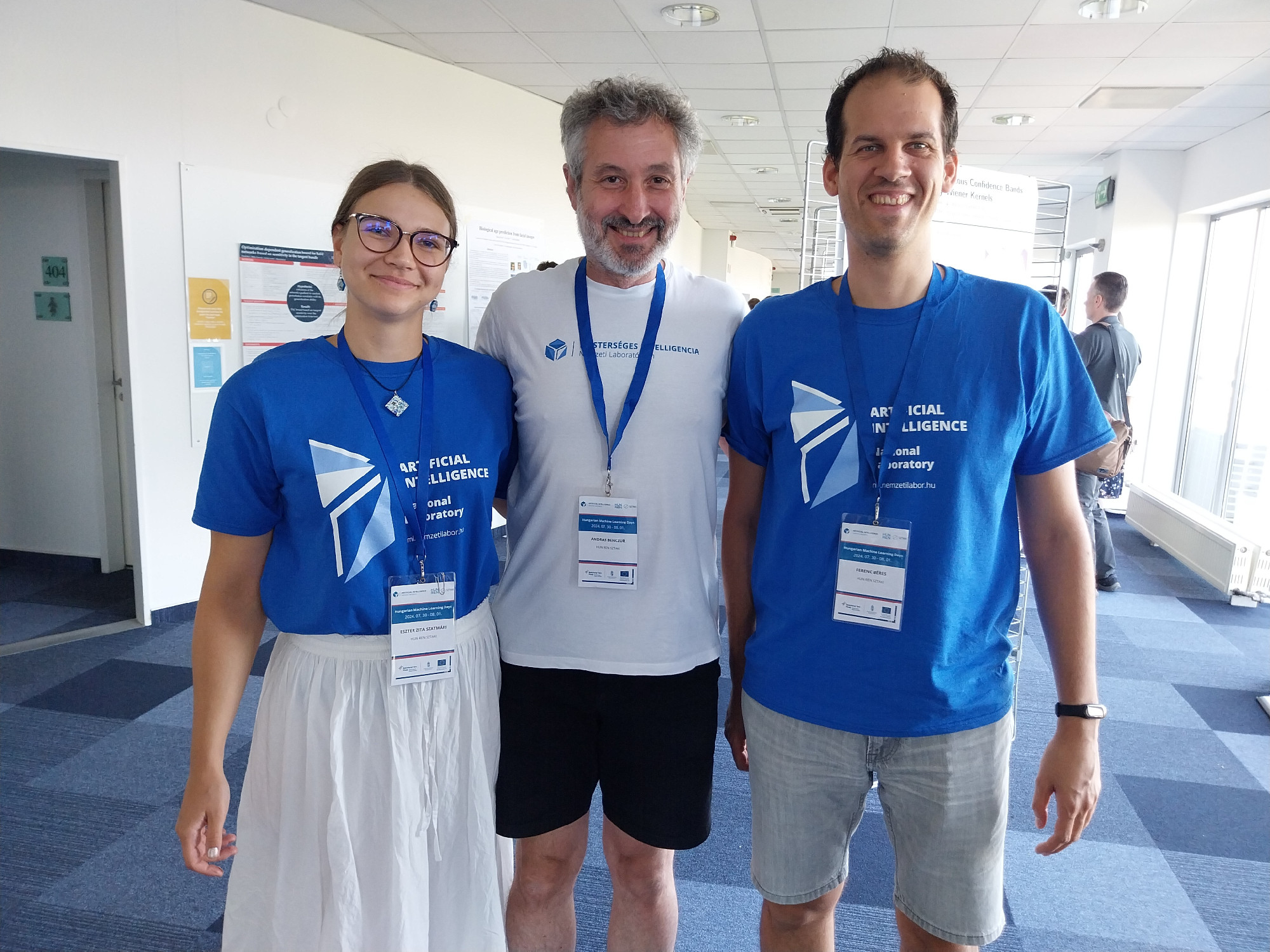
Our Committed Team
Many professions are predicted to disappear due to the rise of AI, but some argue that AI implementation can also create new job opportunities. In this context, András Benczúr notes that, so far, the demand for AI developers has increased faster than job losses. Automation is very costly: while large language models make many tasks easier, they still require human intervention. Similarly, self-driving cars have not entirely eliminated the need for taxi or truck drivers, as once promised, but road safety has been greatly improved by technological advancements such as adaptive cruise control, lane departure warning, and pedestrian detection systems.
When we asked whether there is a physical limit to the server farms supporting AI computations—such as whether supercomputers could consume the energy supply of an entire country—the head of the SZTAKI Artificial Intelligence Laboratory explained: "We have observed the immense energy consumption associated with training large language models. These processes have largely reached the limits of data and model sizes, and there is currently no evidence that significantly larger training capacities can be effectively utilised. The energy demands of current models are indeed very high, and reducing this while maintaining model quality remains a crucial research topic."
According to András Benczúr, one of the key conclusions from the discussions at the meeting was that large language models are far from solving all problems. Many open questions remain, such as error guarantees in reinforcement learning, theories on the generalisation capabilities of large neural networks, and various unresolved issues in bioinformatics applications. The meeting also addressed concerns about model susceptibility to deception, including adversarial examples, data poisoning in training datasets, and the issue of undetectable software backdoors that could trigger malicious behaviour.
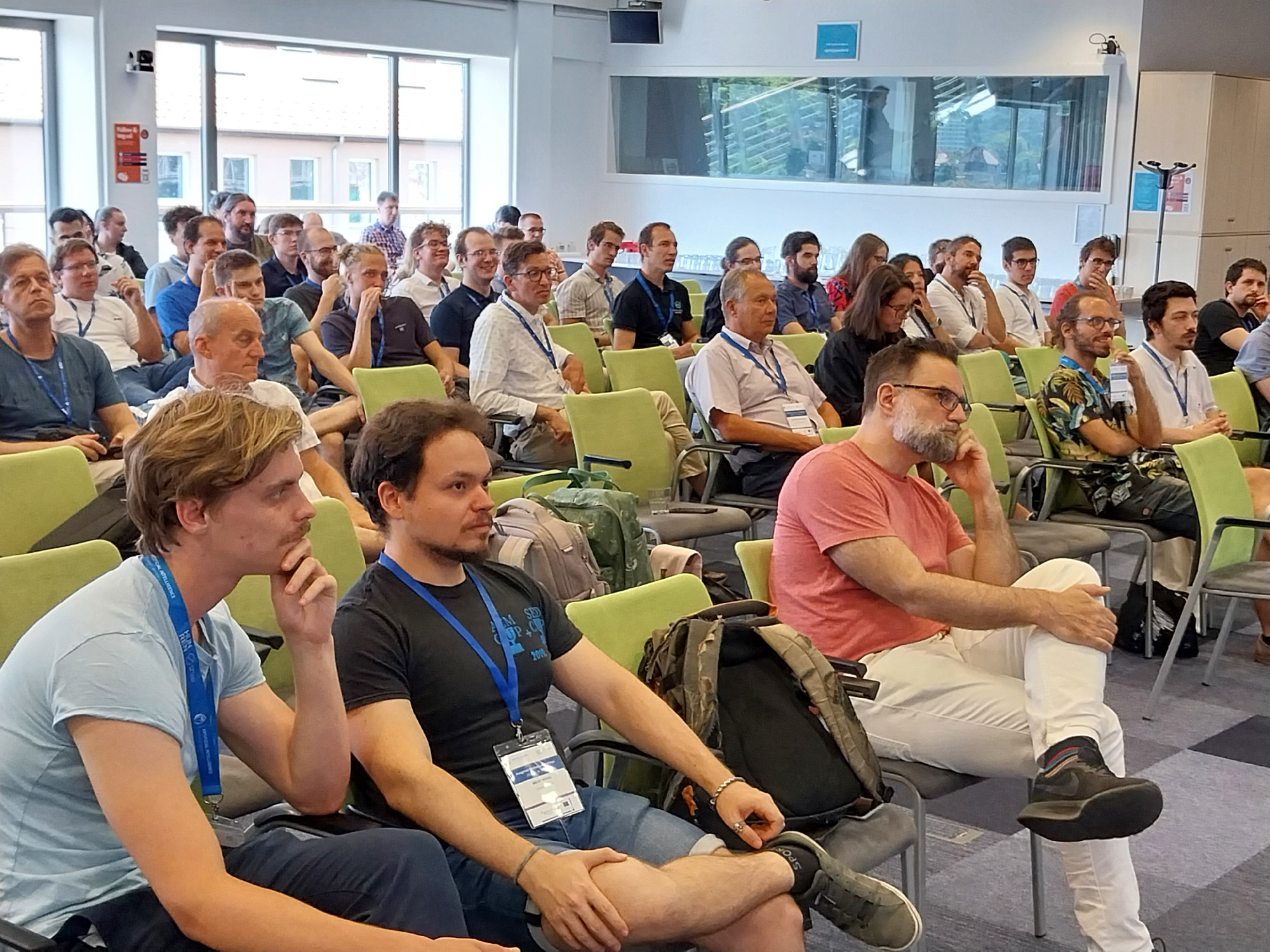
Among machine learning models, supervised learning involves training the machine using examples provided by developers, while unsupervised learning allows the machine to autonomously study typically unstructured input data. We asked if there are any dangers associated with this approach. According to the researcher, extending reinforcement learning with error bounds aims to ensure that self-learning systems do not enter states during the learning process that could compromise the safety of the system or its environment. Currently, this is a very active area of research.